AI-RESCUE
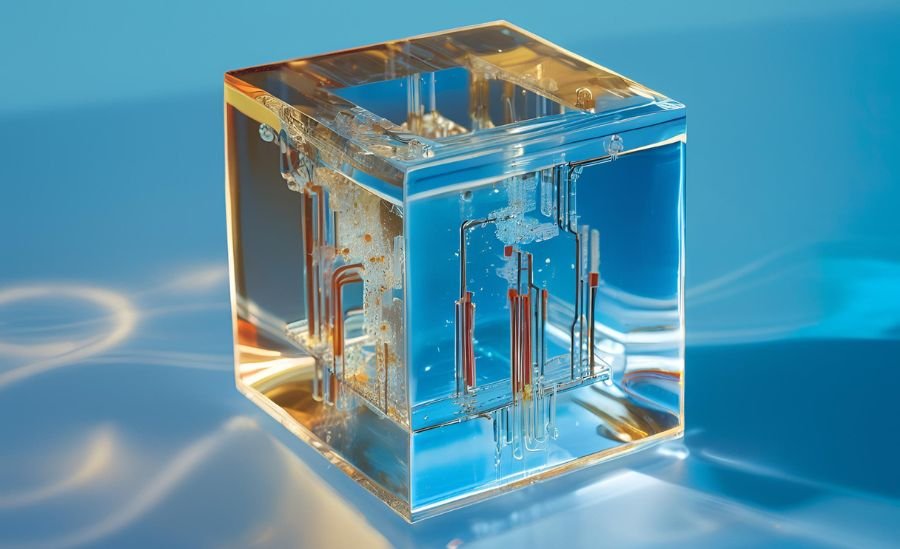
Responsible:
NRRP
DEIB Role: Partner
Start date: 2024-05-01
Length: 21 months
Project abstract
The AI-RESCUE project stems from the increasing relevance of Artificial Intelligence, particularly Deep Learning, in critical applications where security and reliability are paramount. The motivation driving this project is the urgent need to understand and mitigate the vulnerabilities of AI systems when deployed in adversarial settings, where malicious entities may attempt to deceive or manipulate these systems. The project aligns with the broader objectives of the SOS AI consortium, especially tasks focused on foundational research and practical experimentation aimed at securing AI systems.
AI-RESCUE aims to extend the SOS AI framework by exploring AI security across three strategically selected domains: multimedia forensics, fraud and anomaly detection in critical systems (especially financial), and neuro-symbolic goal recognition. These areas are chosen for their societal relevance and the unique challenges they present in terms of adversarial threats.
The project is organized into three main work packages reflecting both theoretical research and applied experimentation. Task 1.3 focuses on foundational security aspects in each domain, including threat modeling, adversarial learning, and the robustness of AI-based detectors. Task 2.3 develops and validates defensive tools and strategies, using real-world scenarios to test the proposed techniques under various adversarial settings, such as white-box, grey-box, and black-box attacks. Finally, Task 3.3 synthesizes the insights gained to produce a comprehensive guide of best practices for the secure application of AI in critical domains.
The implementation plan emphasizes interdisciplinary collaboration across a consortium of five academic institutions, each contributing complementary expertise—from media security and AI theory to cybersecurity and automated reasoning. The project adopts a rigorous methodology that combines formal theoretical modeling, adversarial training techniques, online learning frameworks, and neuro-symbolic systems. Experimental campaigns are planned to evaluate the efficacy of defense mechanisms, guided by real-world data and realistic threat scenarios.
AI-RESCUE ultimately aspires to not only advance the scientific understanding of adversarial AI but also provide tangible tools and guidelines that can be adopted by practitioners, thereby contributing to the development of robust, secure, and trustworthy AI technologies.
AI-RESCUE aims to extend the SOS AI framework by exploring AI security across three strategically selected domains: multimedia forensics, fraud and anomaly detection in critical systems (especially financial), and neuro-symbolic goal recognition. These areas are chosen for their societal relevance and the unique challenges they present in terms of adversarial threats.
The project is organized into three main work packages reflecting both theoretical research and applied experimentation. Task 1.3 focuses on foundational security aspects in each domain, including threat modeling, adversarial learning, and the robustness of AI-based detectors. Task 2.3 develops and validates defensive tools and strategies, using real-world scenarios to test the proposed techniques under various adversarial settings, such as white-box, grey-box, and black-box attacks. Finally, Task 3.3 synthesizes the insights gained to produce a comprehensive guide of best practices for the secure application of AI in critical domains.
The implementation plan emphasizes interdisciplinary collaboration across a consortium of five academic institutions, each contributing complementary expertise—from media security and AI theory to cybersecurity and automated reasoning. The project adopts a rigorous methodology that combines formal theoretical modeling, adversarial training techniques, online learning frameworks, and neuro-symbolic systems. Experimental campaigns are planned to evaluate the efficacy of defense mechanisms, guided by real-world data and realistic threat scenarios.
AI-RESCUE ultimately aspires to not only advance the scientific understanding of adversarial AI but also provide tangible tools and guidelines that can be adopted by practitioners, thereby contributing to the development of robust, secure, and trustworthy AI technologies.