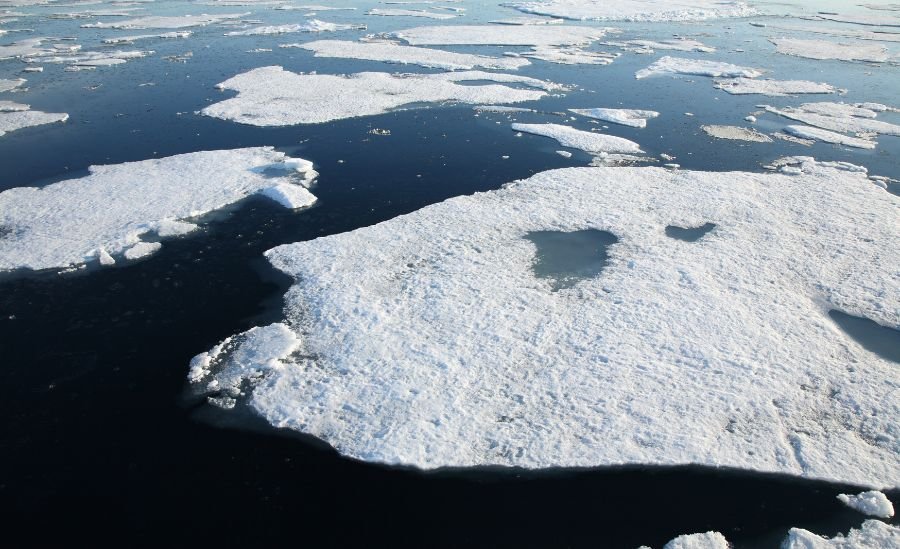
The dynamics of the Arctic region are strongly linked to those of climate change: warming in the Arctic is four times faster than the global average, and some of the phenomena there have large-scale climatic and socioeconomic influences. Currently, however, the causes of these phenomena are largely unknown, and an approach based solely on traditional modeling, which requires identifying the mathematical relationships underlying the physical processes, does not seem to allow for a comprehensive analysis.
The MELTED (MachinE Learning for arcTic ice prEDiction) project aims to improve the understanding of processes related to Arctic ice dynamics by integrating traditional modeling with advanced Data Assimilation and Machine Learning methodologies. Indeed, these techniques are capable of processing huge masses of data, often incomplete (data sparseness), and bringing to light complex relationships not represented in available physical models (interpretability). The combination of the two approaches is therefore intended to address some of the problems that characterize traditional modeling, whose effectiveness is currently limited by poor knowledge of many physical processes and high computational demands.
To achieve these goals, MELTED's first task is to collect observed and reanalysis data for the Arctic region, and perform preliminary processing. These data are then to be processed using advanced Machine Learning techniques. In particular, the MELTED project aspires to correct systematic errors that affect physically based models, thereby improving our ability to predict the dynamics of Arctic ice.