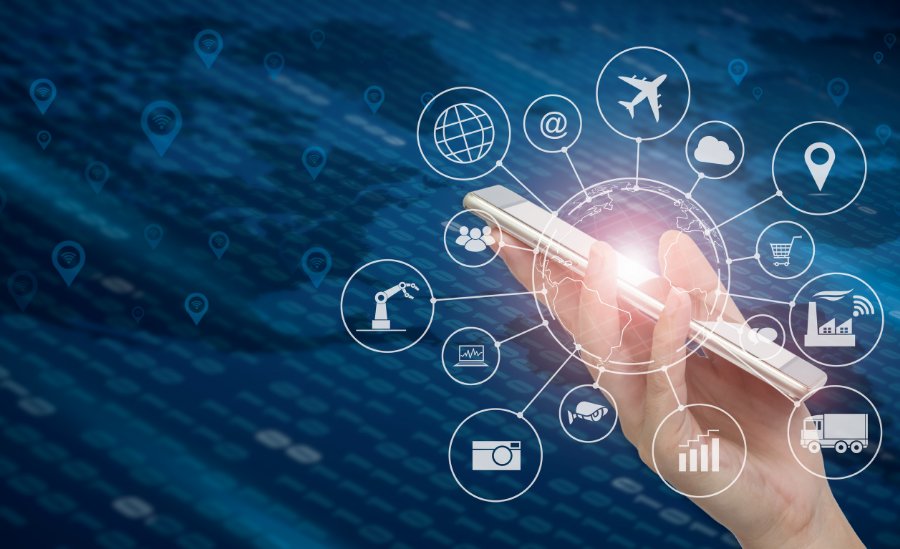
Research Lines:
Internet of Things (IoT) devices already pervade our lives and by 2025 we will have four IoT devices per person on average. Things are huge data generators and require that efficient, reliable, and secure solutions be conceived for their management. Machine Learning (ML) is increasingly selected as an enabler since it can help tame the complexity of these systems, identify recurring patterns, detect anomalies, and make decisions.
Project EMELIOT (Engineered MachinE Learning-intensive IoT systems) studies solutions for engineering highly-dependable, ML-intensive IoT systems. EMELIOT foresees systems where different sensors collect data from physical systems, which in turn can get their status changed by actuators. Collected data are filtered, aggregated, and used to manage ML models locally, on the edge, by dedicated IoT gateways, or on remote cloud servers.
This flexibility allows for diverse solutions and can take care of different processes - responsibility, latency issues, and privacy concerns - since the amount of data to move can be adjusted and data can be analyzed locally. The overarching goal of the project is to provide software engineers, data scientists, and ML experts a comprehensive set of methodologies, solutions, and tools to improve the development, verification, and operation of ML-intensive IoT systems.