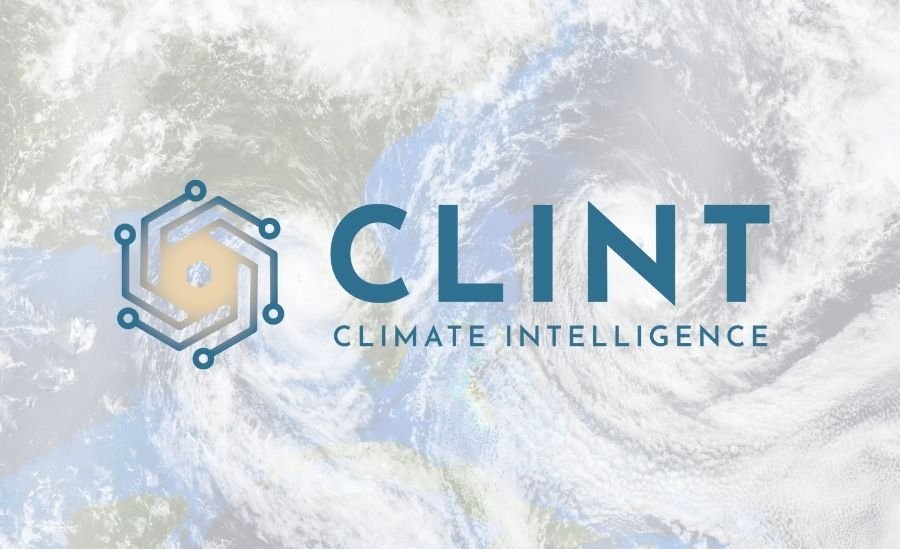
Weather and climate extremes pose challenges for adaptation and mitigation policies and disaster risk management, emphasizing the Climate Services' value in supporting strategic decision-making. Today, Climate Services can benefit from an unprecedented availability of data, particularly from the Copernicus Climate Change Service, and from recent advances in Artificial Intelligence (AI) to exploit these data's full potential. The main objective of CLINT is the development of an AI framework composed of Machine Learning (ML) techniques and algorithms to process big climate datasets for improving Climate Science in the detection, causation, and attribution of Extreme Events, including tropical cyclones, heatwaves, and warm nights, and extreme droughts, along with compound events and concurrent extremes.
Specifically, the framework will support (1) the detection of spatial and temporal patterns and evolutions of climatological fields associated with Extreme Events, (2) the validation of the physically-based nature of causality discovered by ML algorithms, and (3) the attribution of past and future Extreme Events to emissions of greenhouse gases and another anthropogenic forcing.
The framework will also cover Extreme Events' quantification impacts on various socio-economic sectors under historical, forecasted, and projected climate conditions by developing innovative and sectorial AI-enhanced Climate Services. These will be demonstrated across different spatial scales, from the pan European scale to support EU policies addressing the Water-Energy-Food Nexus to the local scale in three types of
Climate Change Hotspots. Finally, according to the most advanced open data and software standards by Climate Services Information Systems, these services will be operationalized into Web Processing Services and into a Demonstrator to facilitate the uptake of project results by public and private entities research and Climate Services development.