Learning-based control of uncertain nonlinear systems: the view from multi-task learning
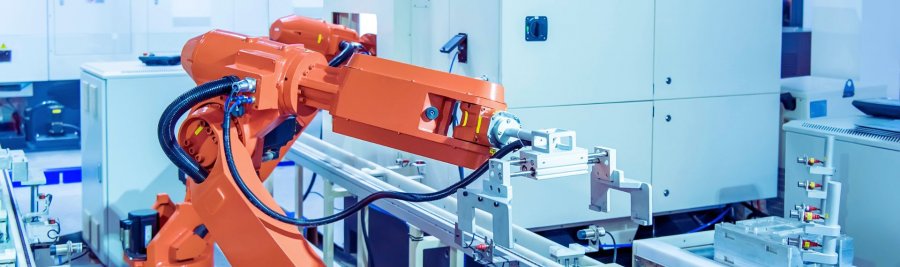
Mercoledì 27 novembre 2024 | 15:00
Dipartimento di Elettronica, Informazione e Bioingegneria - Politecnico di Milano
Sala Conferenze "Emilio Gatti" (Edificio 20)
Dipartimento di Elettronica, Informazione e Bioingegneria - Politecnico di Milano
Sala Conferenze "Emilio Gatti" (Edificio 20)
Sommario
Model-based control has established itself as the prime framework to perform certifiably-safe regulation of dynamical systems. In its original set-up, such a methodology relies on first-principles-models of the plant — and these, due to the increasing complexity of modern systems, can be inadequate or missing.
To cope with this issue, the growing availability of data paved the way to learning-based control design, where system identification is deployed to obtain a model of the system’s response to external inputs. In this framework, system estimates are also often complemented with uncertainty bounds around them, thus enabling the design of robust or stochastic control schemes.
In the first part of this talk, Dr. Anna Scampicchio will give her perspective on learning-based control, sketching her research journey from (kernel-based) identification to control —and back. Next, she will provide a line of work connecting identification and control from the viewpoint of multi-task learning. Specifically, she will start by showing an innovative solution to jointly estimate multiple functions under orthogonality constraints, exploiting the stochastic interpretation of kernel-based methods to overcome the non-convexity of the deterministic formulation.
Then, since the general nonparametric set-up is encumbered by its high computational complexity, she will build upon the so-called sparse-spectrum approximation and tailor it to the multi-task setting. Finally, she will show the effectiveness of the resulting model through experiments on hardware, where a learning-based model predictive controller is successfully implemented on real-world robotic systems.
To cope with this issue, the growing availability of data paved the way to learning-based control design, where system identification is deployed to obtain a model of the system’s response to external inputs. In this framework, system estimates are also often complemented with uncertainty bounds around them, thus enabling the design of robust or stochastic control schemes.
In the first part of this talk, Dr. Anna Scampicchio will give her perspective on learning-based control, sketching her research journey from (kernel-based) identification to control —and back. Next, she will provide a line of work connecting identification and control from the viewpoint of multi-task learning. Specifically, she will start by showing an innovative solution to jointly estimate multiple functions under orthogonality constraints, exploiting the stochastic interpretation of kernel-based methods to overcome the non-convexity of the deterministic formulation.
Then, since the general nonparametric set-up is encumbered by its high computational complexity, she will build upon the so-called sparse-spectrum approximation and tailor it to the multi-task setting. Finally, she will show the effectiveness of the resulting model through experiments on hardware, where a learning-based model predictive controller is successfully implemented on real-world robotic systems.
Biografia
Anna Scampicchio is a postdoctoral researcher at the Institute for Dynamic Systems and Control of ETH Zurich. She received in 2015 the Bachelor degree in Information Engineering and in 2017 the Masters degree in Automation Engineering, both cum laude, from the University of Padova.
In 2017 she was awarded with the Roberto Rocca scholarship for her career during the Masters Degree. She held a visiting position at the Department of Applied Mathematics of University of Washington, Seattle, in 2019. In 2021 she received the Ph.D. in Information Engineering from the University of Padova. Her research interests lie at the interplay among system identification, machine learning and control design.
In 2017 she was awarded with the Roberto Rocca scholarship for her career during the Masters Degree. She held a visiting position at the Department of Applied Mathematics of University of Washington, Seattle, in 2019. In 2021 she received the Ph.D. in Information Engineering from the University of Padova. Her research interests lie at the interplay among system identification, machine learning and control design.