Wasserstein Distributionally Robust Bayesian Optimization: Regret bounds for black-box functions' optimization under context distribution uncertainty
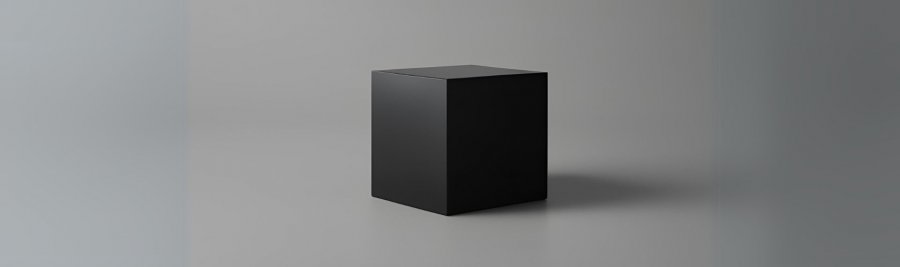
22 Aprile 2025 | 14:00
Sala Conferenze Emilio Gatti
Edificio 20
Speaker: Francesco Micheli (ETH Zurich)
Contatti: Prof. Simone Garatti | simone.garatti@polimi.it
Sala Conferenze Emilio Gatti
Edificio 20
Speaker: Francesco Micheli (ETH Zurich)
Contatti: Prof. Simone Garatti | simone.garatti@polimi.it
Sommario
In this talk we introduce Wasserstein Distributionally Robust Bayesian Optimization (WDRBO), a novel algorithm for sequential decision-making under context distributional uncertainty. WDRBO tackles the challenge of optimizing black-box objective functions in the presence of uncontrollable contextual variables whose distributions are uncertain. By modelling the context distributional uncertainty using Wasserstein ambiguity sets and exploiting Lipschitz bounds on the surrogate model, WDRBO can handle continuous context distributions while maintaining computational tractability. Our theoretical analysis combines recent results in self-normalized concentration in Hilbert spaces and finite-sample bounds for distributionally robust optimization to establish sublinear regret bounds that match state-of-the-art results. Through comprehensive empirical evaluation on both synthetic and real-world problems, we demonstrate that WDRBO achieves competitive performance with significantly lower computational complexity compared to existing distributionally robust approaches.