NECSTFridayTalk – From Data Privacy to Performance: AI Accelerators for Healthcare Federated Learning
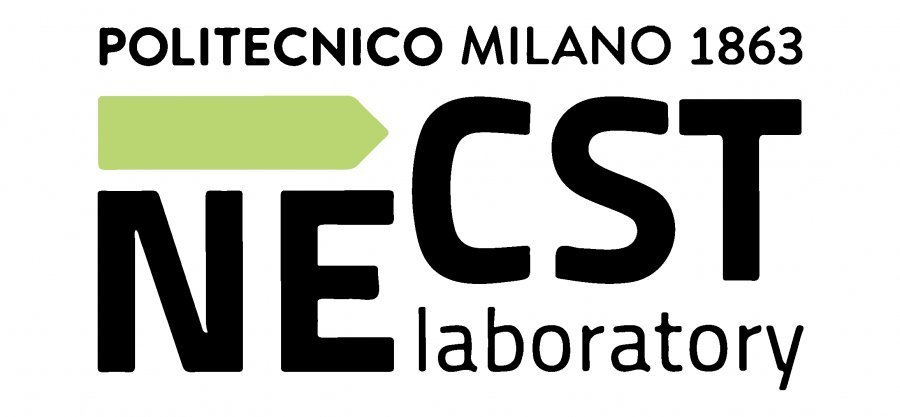
Presenter: Giuseppe Sorrentino
PHD Student in Information Technology
DEIB - NECSTLab Meeting Room (Bld. 20)
Online by Zoom
February 28 th, 2025 | 11.30 am
Contact: Prof. Marco Santambrogio
PHD Student in Information Technology
DEIB - NECSTLab Meeting Room (Bld. 20)
Online by Zoom
February 28 th, 2025 | 11.30 am
Contact: Prof. Marco Santambrogio
Sommario
On February 28 th, 2025 at 11.30 am a new appointment of NECSTFridayTalk series titled "From Data Privacy to Performance: AI Accelerators for Healthcare Federated Learning" will take place at DEIB NECSTLab Meeting Room (Building 20) and on line by Zoom.
During this talk, we will have, as speaker, Giuseppe Sorrentino, PhD student at Dipartimento di Elettronica, Informazione e Bioingegneria.
Modern AI accelerators are transforming the technological landscape, delivering the performance required to enhance Deep Learning (DL) and Machine Learning (ML) workloads across various fields, including robotics, computer vision, and healthcare. In healthcare, DL models offer high accuracy but require processing vast amounts of sensitive data, raising significant privacy concerns. Federated learning addresses this challenge by enabling secure model training without sharing raw data, but it introduces substantial computational overhead. In this talk, I will present my research on reducing this overhead by leveraging heterogeneous AI systems, optimizing each stage of the federated learning pipeline through efficient hardware acceleration. As a use case, I focus on non-rigid image registration, a compute-intensive DL task that exemplifies both the challenges of healthcare federated learning systems and the potential of heterogeneous AI accelerators.
During this talk, we will have, as speaker, Giuseppe Sorrentino, PhD student at Dipartimento di Elettronica, Informazione e Bioingegneria.
Modern AI accelerators are transforming the technological landscape, delivering the performance required to enhance Deep Learning (DL) and Machine Learning (ML) workloads across various fields, including robotics, computer vision, and healthcare. In healthcare, DL models offer high accuracy but require processing vast amounts of sensitive data, raising significant privacy concerns. Federated learning addresses this challenge by enabling secure model training without sharing raw data, but it introduces substantial computational overhead. In this talk, I will present my research on reducing this overhead by leveraging heterogeneous AI systems, optimizing each stage of the federated learning pipeline through efficient hardware acceleration. As a use case, I focus on non-rigid image registration, a compute-intensive DL task that exemplifies both the challenges of healthcare federated learning systems and the potential of heterogeneous AI accelerators.
The NECSTLab is a DEIB laboratory, with different research lines on advanced topics in computing systems: from architectural characteristics, to hardware-software codesign methodologies, to security and dependability issues of complex system architectures.
Every week, the “NECSTFridayTalk” invites researchers, professionals or entrepreneurs to share their work experiences and projects they are implementing in the “Computing Systems”.