On the Learnability and Optimality of Graph Deep Learning Models for Time Series Analysis
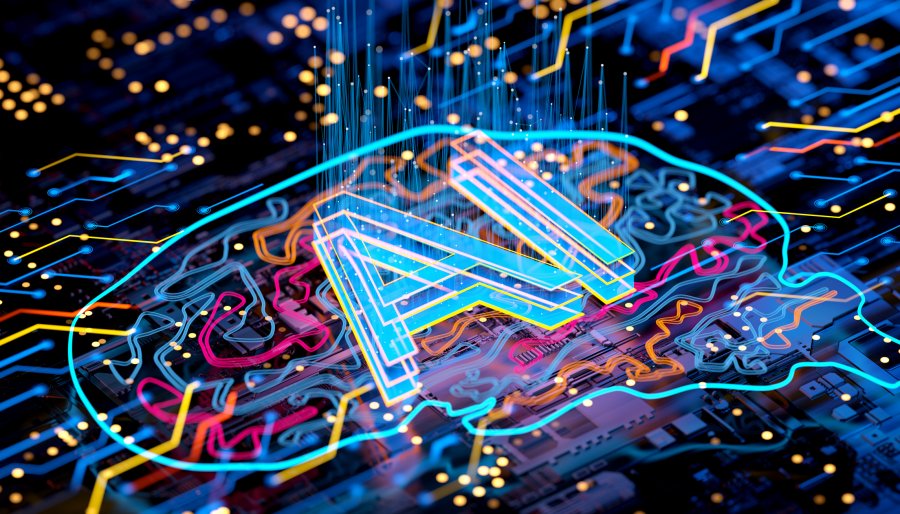
Presenter: Daniele Zambon
Dalle Molle Institute for Artificial Intelligence (IDSIA)
Università della Svizzera italiana
DEIB - Conference Room "E. Gatti" (Bld. 20)
April 8th, 2025 | 4.00 pm
Contact: Prof. Manuel Roveri
Dalle Molle Institute for Artificial Intelligence (IDSIA)
Università della Svizzera italiana
DEIB - Conference Room "E. Gatti" (Bld. 20)
April 8th, 2025 | 4.00 pm
Contact: Prof. Manuel Roveri
Sommario
On April 8th, 2025 at 4.00 pm the seminar titled "On the learnability and optimality of graph deep learning models for time series analysis" will take place at DEIB Conference Room "Emilio Gatti" (Building 20).
Graph deep learning is redefining the analysis of time series across diverse domains such as environmental monitoring, smart cities, and finance. Unlike traditional methods, this framework leverages functional dependencies between time series to provide more accurate predictions. In this seminar, I will first provide an overview of graph deep learning for time series processing. Then, I will present two of our recent contributions to the development of scalable and reliable models. The first introduces a novel method for evaluating model optimality and identifying areas for improvement. The second addresses the learnability from data of the graph that connects the time series – an essential but often unavailable piece of information.
Graph deep learning is redefining the analysis of time series across diverse domains such as environmental monitoring, smart cities, and finance. Unlike traditional methods, this framework leverages functional dependencies between time series to provide more accurate predictions. In this seminar, I will first provide an overview of graph deep learning for time series processing. Then, I will present two of our recent contributions to the development of scalable and reliable models. The first introduces a novel method for evaluating model optimality and identifying areas for improvement. The second addresses the learnability from data of the graph that connects the time series – an essential but often unavailable piece of information.
Biografia
Daniele Zambon is a postdoctoral researcher at the Dalle Molle Institute for Artificial Intelligence (IDSIA), affiliated with Università della Svizzera italiana (USI) in Switzerland.
He is a member of the IEEE Task Force on Learning for Structured Data and the Graph Machine Learning Group in Lugano. His research focuses on graph representation learning, learning in non-stationary environments, and time series analysis. He graduated with honors from the University of Milan (IT) with a degree in Mathematics and earned a PhD in Informatics from USI. He has been visiting researcher at the University of Florida (USA) and the University of Exeter (UK). His work has been published in top-tier journals and conferences in the field, including JMLR, IEEE TPAMI, IEEE TNNLS, IEEE TSP, NeurIPS, ICLR, and ICML.
He is a member of the IEEE Task Force on Learning for Structured Data and the Graph Machine Learning Group in Lugano. His research focuses on graph representation learning, learning in non-stationary environments, and time series analysis. He graduated with honors from the University of Milan (IT) with a degree in Mathematics and earned a PhD in Informatics from USI. He has been visiting researcher at the University of Florida (USA) and the University of Exeter (UK). His work has been published in top-tier journals and conferences in the field, including JMLR, IEEE TPAMI, IEEE TNNLS, IEEE TSP, NeurIPS, ICLR, and ICML.
Website: https://dzambon.github.io/ Email: daniele.zambon@usi.ch