NECSTFridayTalk – Tarallo: Evading Behavioral Malware Detectors in the Problem Space
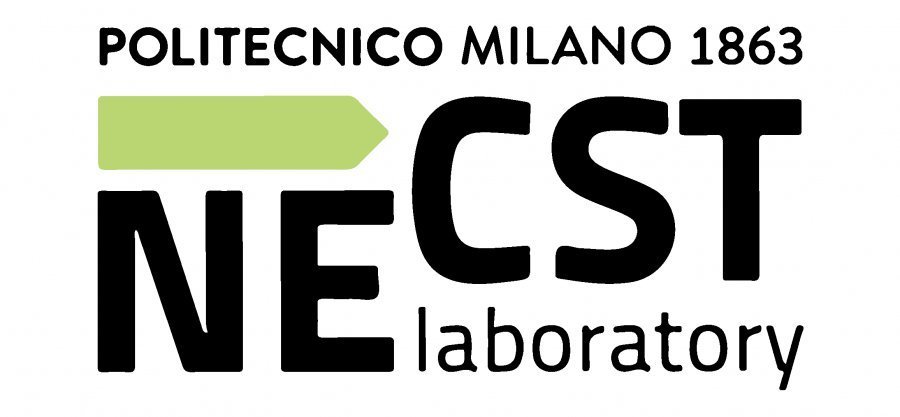
Presenter: Gabriele Digregorio
PHD Student in Information Technology
DEIB - NECSTLab Meeting Room (Bld. 20)
Online by Zoom
December 13th, 2024 | 11.30 am
Contact: Prof. Marco Santambrogio
PHD Student in Information Technology
DEIB - NECSTLab Meeting Room (Bld. 20)
Online by Zoom
December 13th, 2024 | 11.30 am
Contact: Prof. Marco Santambrogio
Sommario
On December 13th, 2024 at 11.30 am a new appointment of NECSTFridayTalk series titled "Tarallo: Evading Behavioral Malware Detectors in the Problem Space" will take place at DEIB NECSTLab Meeting Room (Building 20) and on line by Zoom.
During this talk, we will have, as speaker, Gabriele Digregorio, PhD Student at Dipartimento di Elettronica, Informazione e Bioingegneria.
Machine learning algorithms can effectively classify malware through dynamic behavior but are susceptible to adversarial attacks. Existing attacks, however, often fail to find an effective solution in both the feature and problem spaces. This issue arises from not addressing the intrinsic nondeterministic nature of malware, namely executing the same sample multiple times may yield significantly different behaviors. Hence, the perturbations computed for a specific behavior may be ineffective for others observed in subsequent executions. We show how an attacker can augment their chance of success by leveraging a new and more efficient feature space algorithm for sequential data, which we have named Position Sensitive - Fast Gradient Sign Method, and by adopting two problem space strategies specially tailored to address nondeterminism in the problem space. We implement our novel algorithm and attack strategies in Tarallo, an end-to-end adversarial framework that significantly outperforms previous works in both white and blackbox scenarios. Our preliminary analysis in a sandboxed environment and against two Recurrent Neural Network (RNN)-based malware detectors, shows that Tarallo achieves a success rate up to 99% on both feature and problem space attacks while significantly minimizing the number of modifications required for misclassification.
During this talk, we will have, as speaker, Gabriele Digregorio, PhD Student at Dipartimento di Elettronica, Informazione e Bioingegneria.
Machine learning algorithms can effectively classify malware through dynamic behavior but are susceptible to adversarial attacks. Existing attacks, however, often fail to find an effective solution in both the feature and problem spaces. This issue arises from not addressing the intrinsic nondeterministic nature of malware, namely executing the same sample multiple times may yield significantly different behaviors. Hence, the perturbations computed for a specific behavior may be ineffective for others observed in subsequent executions. We show how an attacker can augment their chance of success by leveraging a new and more efficient feature space algorithm for sequential data, which we have named Position Sensitive - Fast Gradient Sign Method, and by adopting two problem space strategies specially tailored to address nondeterminism in the problem space. We implement our novel algorithm and attack strategies in Tarallo, an end-to-end adversarial framework that significantly outperforms previous works in both white and blackbox scenarios. Our preliminary analysis in a sandboxed environment and against two Recurrent Neural Network (RNN)-based malware detectors, shows that Tarallo achieves a success rate up to 99% on both feature and problem space attacks while significantly minimizing the number of modifications required for misclassification.
The NECSTLab is a DEIB laboratory, with different research lines on advanced topics in computing systems: from architectural characteristics, to hardware-software codesign methodologies, to security and dependability issues of complex system architectures.
Every week, the “NECSTFridayTalk” invites researchers, professionals or entrepreneurs to share their work experiences and projects they are implementing in the “Computing Systems”.