Flexible and adaptive AI for wireless transceivers
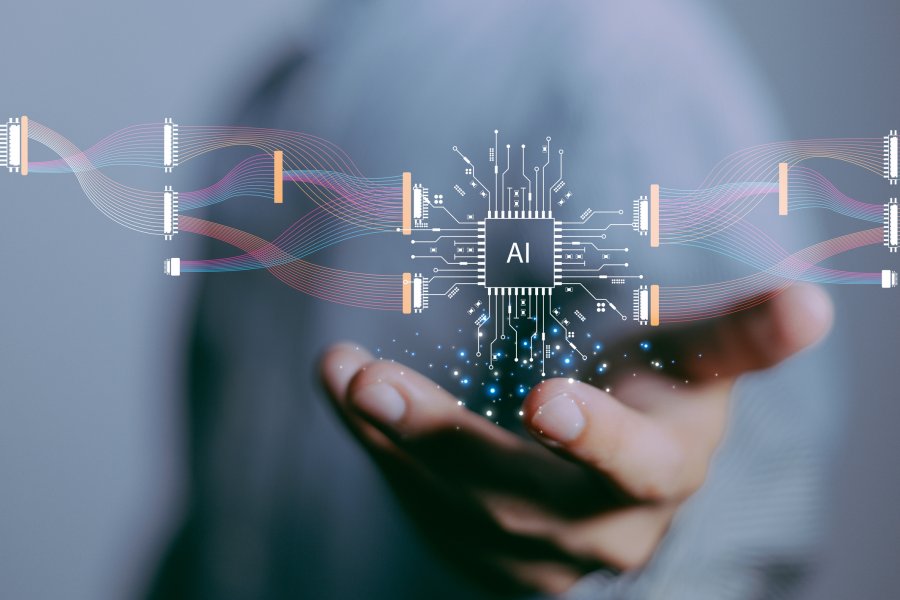
Speaker: Prof. Nir Shlezinger
Ben-Gurion University of the Negev, Israel
DEIB - Carlo Erba Room (Bld. 7)
Piazza Leonardo da Vinci, 32
September 9th, 2024 | 11.00 am
Contact: Prof. Marco Mezzavilla
Research Line: Information transmission
Ben-Gurion University of the Negev, Israel
DEIB - Carlo Erba Room (Bld. 7)
Piazza Leonardo da Vinci, 32
September 9th, 2024 | 11.00 am
Contact: Prof. Marco Mezzavilla
Research Line: Information transmission
Sommario
On September 9th, 2024 at 11.00 am the seminar "Flexible and adaptive AI for wireless transceivers" will take place at DEIB Carlo Erba Room (Building 7).
Artificial intelligence (AI) is envisioned to play a key role in future wireless technologies, with deep neural networks (DNNs) enabling digital receivers to learn to operate in challenging communication scenarios. However, wireless transceiver design poses unique challenges that fundamentally differ from those encountered in traditional deep learning domains. The main challenges arise from the limited power and computational resources of wireless devices, as well as from the dynamic nature of wireless communications, which causes continual changes to the data distribution. These challenges impair conventional AI based on highly-parameterized DNNs, motivating the development of adaptive, flexible, and light-weight AI for wireless communications, which is the focus of this talk. We will discuss how AI-based design of wireless receivers requires rethinking of the three main pillars of AI: architecture, data, and training algorithms. In terms of architecture, we will review how to design compact DNNs via model-based deep learning. Then, we will discuss how to acquire training data for deep receivers without compromising spectral efficiency. Finally, we will review efficient, reliable, and robust training algorithms which enhance existing deep learning techniques via meta-learning and generalized Bayesian learning, as well as propose different learning approach based on modelling learning dynamics as a state space model.
Nir Shlezinger is an assistant professor in the School of Electrical and Computer Engineering at Ben-Gurion University, Israel. He received his B.Sc., M.Sc., and Ph.D. degrees in 2011, 2013, and 2017, respectively, from Ben-Gurion University, Israel, all in electrical and computer engineering. From 2017 to 2019, he was a postdoctoral researcher at the Technion, and from 2019 to 2020, he was a postdoctoral researcher at the Weizmann Institute of Science, where he was awarded the FGS Prize for outstanding research achievements. His research interests include communications, information theory, signal processing, and machine learning.
Artificial intelligence (AI) is envisioned to play a key role in future wireless technologies, with deep neural networks (DNNs) enabling digital receivers to learn to operate in challenging communication scenarios. However, wireless transceiver design poses unique challenges that fundamentally differ from those encountered in traditional deep learning domains. The main challenges arise from the limited power and computational resources of wireless devices, as well as from the dynamic nature of wireless communications, which causes continual changes to the data distribution. These challenges impair conventional AI based on highly-parameterized DNNs, motivating the development of adaptive, flexible, and light-weight AI for wireless communications, which is the focus of this talk. We will discuss how AI-based design of wireless receivers requires rethinking of the three main pillars of AI: architecture, data, and training algorithms. In terms of architecture, we will review how to design compact DNNs via model-based deep learning. Then, we will discuss how to acquire training data for deep receivers without compromising spectral efficiency. Finally, we will review efficient, reliable, and robust training algorithms which enhance existing deep learning techniques via meta-learning and generalized Bayesian learning, as well as propose different learning approach based on modelling learning dynamics as a state space model.
Nir Shlezinger is an assistant professor in the School of Electrical and Computer Engineering at Ben-Gurion University, Israel. He received his B.Sc., M.Sc., and Ph.D. degrees in 2011, 2013, and 2017, respectively, from Ben-Gurion University, Israel, all in electrical and computer engineering. From 2017 to 2019, he was a postdoctoral researcher at the Technion, and from 2019 to 2020, he was a postdoctoral researcher at the Weizmann Institute of Science, where he was awarded the FGS Prize for outstanding research achievements. His research interests include communications, information theory, signal processing, and machine learning.
WebEx: Meeting link:
https://politecnicomilano.webex.com/meet/marco.mezzavilla
Meeting number:
2785 425 9105
Video address:
marco.mezzavilla@politecnicomilano.webex.com