Adaptive Learning-based Model Predictive Control for Uncertain Interconnected Systems
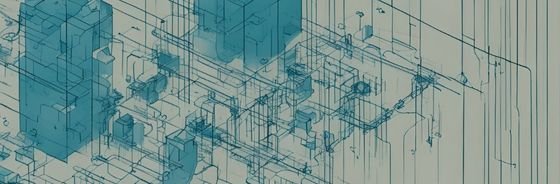
Speaker: Ahmed Aboudonia (ETH Zurich)
Wednesday, June 5, 2024 | 2:00 p.m.
Nicola Schiavoni Seminar Room (Building 20)
Contacts: Prof. Lorenzo Fagiano
Wednesday, June 5, 2024 | 2:00 p.m.
Nicola Schiavoni Seminar Room (Building 20)
Contacts: Prof. Lorenzo Fagiano
Sommario
Control of safety-critical interconnected systems has recently attracted a lot of attention since these systems can depict various infrastructure systems that form the foundation of our society and economy. Model predictive control (MPC) shows significant potential as a viable option to control this class of systems. A primary downside of MPC is the necessity of a precise model which could be hard to acquire and/or complex to utilize. Thus, simplified models are typically employed together with a description of the uncertainty within the MPC framework. In this talk, we present an adaptive learning-based MPC framework comprising a learning phase and an adaptation phase. In the learning phase, a better description of the uncertainty is continually learnt using data collected online. In the adaptation phase, the MPC ingredients are adapted based on the updated uncertainty description. We also show that this scheme yields a better trade-off between closed-loop performance and computation cost when compared to existing schemes including robust, adaptive and learning-based MPC.
Biografia
Ahmed Aboudonia is a postdoctoral researcher with the Automatic Control Laboratory (IfA) at ETH Zurich. He received the PhD degree in electrical engineering from ETH Zurich in 2023, the M.Sc. degree in control engineering from Sapienza University of Rome in 2018 and the B.Sc. degree in mechatronics engineering from the German University in Cairo in 2014. During his studies, he interned and visited Siemens, TU Darmstadt and the German Aerospace Center (DLR). His research interests lie in the intersection of control theory, mathematical optimization and machine learning with application to robotics, energy and transportation systems.