Collaborative decentralized data-driven methods for estimation, monitoring, and diagnostics in the process industry
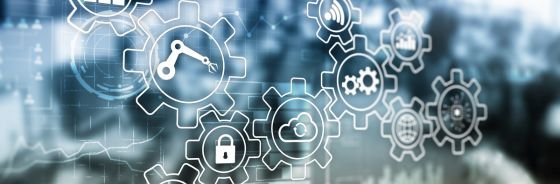
Speaker: Simone Smeraldo (DEIB, Politecnico di Milano)
DEIB - Emilio Gatti Conference Room (Building 20)
May 23, 2024 | 11:45 am
Contacts: Prof. Simone Formentin
Sommario
In extensive industrial contexts, a fleet of similar, yet not identical, assets has to be often optimized and monitored. While the equipment may fundamentally be similar in design, it may differ in size or operate in diverse environment conditions. It is therefore not realistic to train a single, unified model for the entire fleet, while separate models would not benefit from each other commonalities and may suffer from severe sparsity of data (e.g., lack of failure events at a single asset level). To cope with these common challenges in industry, our exploration delved into the Multi-Task Feature Learning (MTFL) literature, where multiple, related models are co-learned simultaneously rather than independently, each using its own data but leveraging useful shared information.