NECSTFridayTalk – Stealthy Adversarial Examples in Computer Vision Models
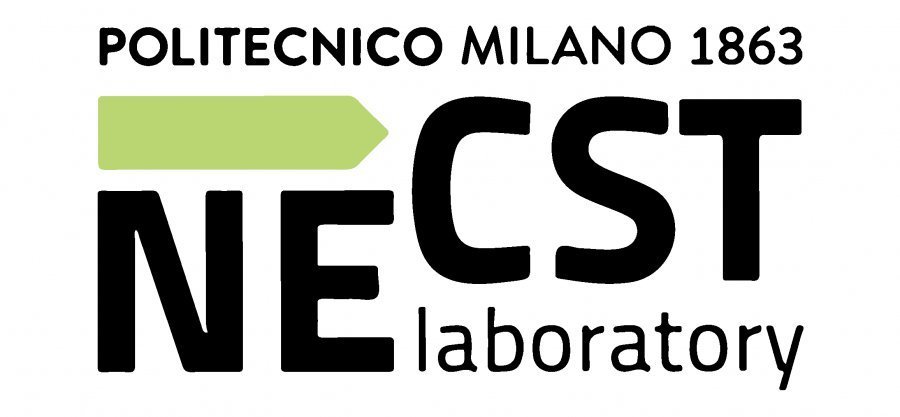
NECSTFridayTalk
Speaker: Francesco Panebianco
DEIB PhD student
DEIB - NECSTLab Meeting Room (Bld. 20)
Online by Zoom
May 17th, 2024 | 11.30 am
Contact: Prof. Marco Santambrogio
Research Line: System architectures
Speaker: Francesco Panebianco
DEIB PhD student
DEIB - NECSTLab Meeting Room (Bld. 20)
Online by Zoom
May 17th, 2024 | 11.30 am
Contact: Prof. Marco Santambrogio
Research Line: System architectures
Sommario
On May 17th, 2024 at 11.30 am a new appointment of NECSTFridayTalk series titled "Stealthy Adversarial Examples in Computer Vision Models" will take place at DEIB NECSTLab Meeting Room (Building 20) and on line by Zoom.
During this talk, we will have as speaker Francesco Panebianco, PhD student in Information Technology at DEIB, Politecnico di Milano on the following about the talk:
Machine Learning has taken hold of every aspect of computer science. While this technology brings significant improvements in terms of performance, it also constitutes a new attack surface. Classifiers and Object Detection Networks are known to be vulnerable to attacks with adversarial examples. These are specially crafted inputs that can purposefully cause misclassification on the victim model. If an adversarial example is submitted in a pipeline, an attacker can exploit this vulnerability to compromise the whole system. We evaluate the strengths of existing black-box solutions in terms of stealthiness to human inspection, stealthiness to automatic detection and robustness to processing. Additionally, we propose ECLIPSE (Evasion of Classifiers with Local Increase in Pixel Sparse Environment), an alternative attack that introduces a tradeoff between these three properties.
During this talk, we will have as speaker Francesco Panebianco, PhD student in Information Technology at DEIB, Politecnico di Milano on the following about the talk:
Machine Learning has taken hold of every aspect of computer science. While this technology brings significant improvements in terms of performance, it also constitutes a new attack surface. Classifiers and Object Detection Networks are known to be vulnerable to attacks with adversarial examples. These are specially crafted inputs that can purposefully cause misclassification on the victim model. If an adversarial example is submitted in a pipeline, an attacker can exploit this vulnerability to compromise the whole system. We evaluate the strengths of existing black-box solutions in terms of stealthiness to human inspection, stealthiness to automatic detection and robustness to processing. Additionally, we propose ECLIPSE (Evasion of Classifiers with Local Increase in Pixel Sparse Environment), an alternative attack that introduces a tradeoff between these three properties.
The NECSTLab is a DEIB laboratory, with different research lines on advanced topics in computing systems: from architectural characteristics, to hardware-software codesign methodologies, to security and dependability issues of complex system architectures.
Every week, the “NECSTFridayTalk” invites researchers, professionals or entrepreneurs to share their work experiences and projects they are implementing in the “Computing Systems”.