Simulated Machine Learning for Personnel Scheduling in Healthcare Management
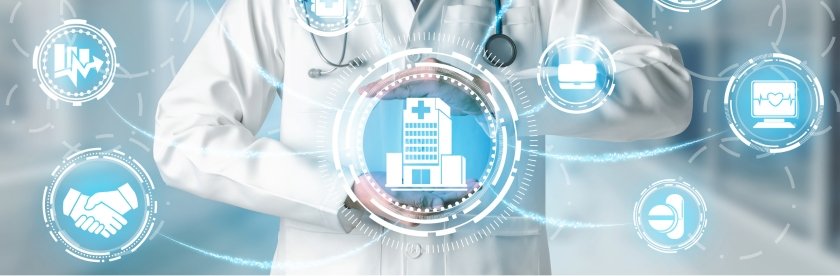
Speaker: Martina Doneda (DEIB, Politecnico di Milano)
DEIB - Nicola Schiavoni Seminar Room (Building 20)
April 24, 2024 | 11:30 pm
Contacts: Prof. Simone Formentin
Sommario
Healthcare is experiencing a surge of interest in the use of Machine Learning (ML) for decision-making. However, employing ML as a silver bullet is a rather naive suggestion. In this work, we make a case for this argument using an example from nurse rostering and rerostering considering absenteeism, referring to instances derived from the Second International Nurse Rostering Competition. On any given day, each nurse can be absent with a certain probability. When such a disruption occurs, the roster needs to be repaired. We assume that we can incorporate some robustness in the roster using reserve shifts. If the reserve shifts cannot repair the disruptions on their own, we assume that ad interim nurses need to be called in, at a high expense. Suppose having a ML model capable of predicting how many nurses will be absent on a given day. How to determine whether using it improves system performance more than using a non-data-driven policy? When using ML to help decision-making, usually i) the ML model is trained and tested, and ii) deployed in several simulated scenarios of the problem to evaluate its effectiveness. We purposely subverted this sequence, simulating the use of a nondescript ML model (an “oracle”) at various levels of predictive performance, deploying it directly in several simulated scenarios. This inversion allows us to evaluate whether it is worth it to invest resources to implement a ML approach, and, be it the case, to determine what its minimal performance needs to be. The effectiveness of the use of such an oracle can then be compared against a set of simpler policies, highlighting how performing the ML model needs to be to outperform them.