Data-Centric AI: Challenges and Research Perspectives for Machine Learning and Deep Learning
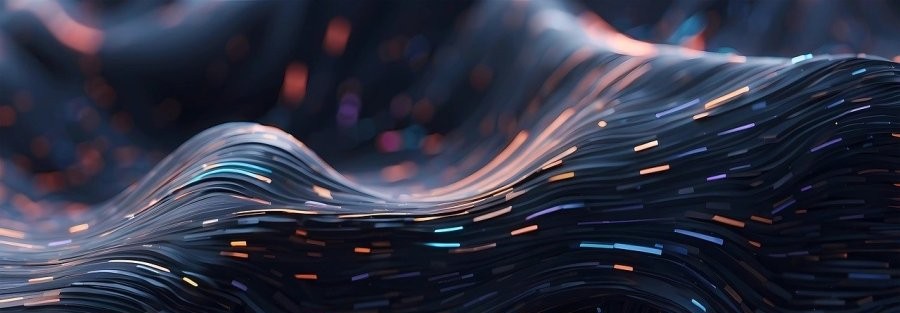
DEIB - PT1 Room (Bld. 20)
May 16th, 2024 | 6.00 pm
Contact: Camilla Sancricca
Research Line: Information systems
May 16th, 2024 | 6.00 pm
Contact: Camilla Sancricca
Research Line: Information systems
Sommario
On May 16th, 2024 at 6.00 pm Camilla Sancricca, PHD Student in Information Technology at Politecnico di Milano, will hold a seminar on "Data-Centric AI: Challenges and Research Perspectives for Machine Learning and Deep Learning" in DEIB PT1 Room (Building 20).
Data-centric AI emphasizes the importance of having high-quality data in AI systems to achieve reliable outcomes. It shifts the focus of such systems to achieve high-quality rather than high-volume data. Traditionally, these systems have focused on optimizing the model's performance, using data only as a static instead of a human-centric, dynamic and evolving input. Data-centric AI is an emerging concept encompassing a range of previous studies on data quality, including human-centered data science. In such systems, data preparation is fundamental to ensure high-quality input data and, thus, guarantee the dependability of the analysis outcomes. This talk aims to introduce the principles of this new research field, highlighting its open challenges, especially in those systems that exploit machine learning and deep learning. Furthermore, it presents a data-centric AI approach to support users in the data preparation pipeline design and the exploration and analysis of the data used in a machine learning pipeline.
Data-centric AI emphasizes the importance of having high-quality data in AI systems to achieve reliable outcomes. It shifts the focus of such systems to achieve high-quality rather than high-volume data. Traditionally, these systems have focused on optimizing the model's performance, using data only as a static instead of a human-centric, dynamic and evolving input. Data-centric AI is an emerging concept encompassing a range of previous studies on data quality, including human-centered data science. In such systems, data preparation is fundamental to ensure high-quality input data and, thus, guarantee the dependability of the analysis outcomes. This talk aims to introduce the principles of this new research field, highlighting its open challenges, especially in those systems that exploit machine learning and deep learning. Furthermore, it presents a data-centric AI approach to support users in the data preparation pipeline design and the exploration and analysis of the data used in a machine learning pipeline.