Abstraction, extraction, and appropriation in the epistemology of ML
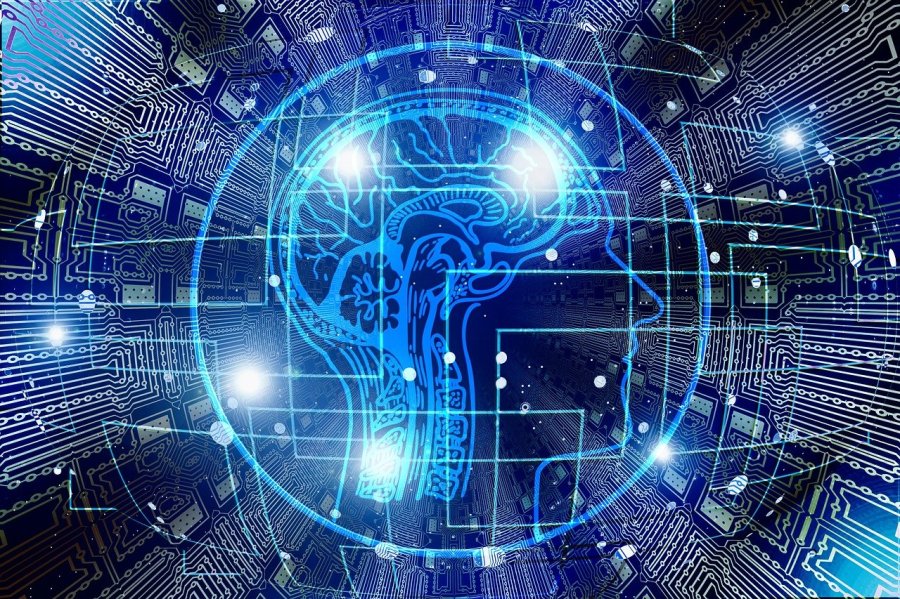
Speaker: Prof. Teresa Numerico
Università Roma Tre
Politecnico di Milano - 26.01 Room (Bld. 26)
Via Golgi, 20
April 18th, 2024 |10.30 am
Contact: Prof. Viola Schiaffonati
Research Line: Artificial intelligence and robotics
Università Roma Tre
Politecnico di Milano - 26.01 Room (Bld. 26)
Via Golgi, 20
April 18th, 2024 |10.30 am
Contact: Prof. Viola Schiaffonati
Research Line: Artificial intelligence and robotics
Sommario
On April 18th, 2024 at 10.30 am the seminar "Abstraction, extraction, and appropriation in the epistemology of ML" will take place at Politecnico di Milano 26.01 Room (Building 26).
In the top-down AI paradigm based on reasoning, all “intelligence” was explicitly programmed into the system through rules and inferential methods, granting programmers full control over the algorithmic organization of AI systems. At every step, the scope of action and implied instructions were transparent. With machine learning techniques this dynamic shifts. “Intelligence” is derived or abstracted through the analysis of vast datasets on which systems are trained. This process also involves data cleaning activities performed by low-key workers and reinforcement learning through human feedback during optimization phases. While humans remain in the loop, they no longer wield complete control over the process. Technical critiques can be directed towards the epistemic criteria employed in processing data and generating results, particularly concerning the induction principle, obscure similarity functions, and necessary discrimination activities inherent in learning biases. These critiques become especially pertinent when results directly impact human rights and significantly influence people's lives. Yet, there’s a broader issue at play. Historically, humans have used tools to support and refine validation criteria for assessing knowledge, but the mediation between the world and our technology, to understand or imagine it, has always been distinctly human. The advent of machine learning techniques and the digitalization of knowledge resources signal a shift in epistemology, where decisions regarding what to abstract from concrete situations to define categories and concepts for judgment, recognition, and creation are no longer solely within human purview. This shift resembles an industrialization of extraction, with digital data serving as the raw material—concretizing human intelligence and the process of searching, recognizing, and sorting relevant content. While machine learning systems heavily rely on human activities, they perceive these activities as objects or exploitable resources, rather than subjects in the abstraction process.
Teresa Numerico is associate professor of Logic and Philosophy of Science at Università Roma Tre. Her publications include: Alan Turing e l’intelligenza delle macchine, (Franco Angeli, 2006), Web Dragons (Morgan Kaufmann, 2007 with M. Gori and I. Witten), L’umanista digitale (Il Mulino, 2010 with D. Fiormonte e F. Tomasi), The Digital Humanist. A critical inquiry (Punctum 2015), Big Data e algoritmi (Carocci, 2021). She works in the history and philosophy of computer science with a focus on the ethical, political and social issues of the digital age and on the epistemology of digital technologies.
In the top-down AI paradigm based on reasoning, all “intelligence” was explicitly programmed into the system through rules and inferential methods, granting programmers full control over the algorithmic organization of AI systems. At every step, the scope of action and implied instructions were transparent. With machine learning techniques this dynamic shifts. “Intelligence” is derived or abstracted through the analysis of vast datasets on which systems are trained. This process also involves data cleaning activities performed by low-key workers and reinforcement learning through human feedback during optimization phases. While humans remain in the loop, they no longer wield complete control over the process. Technical critiques can be directed towards the epistemic criteria employed in processing data and generating results, particularly concerning the induction principle, obscure similarity functions, and necessary discrimination activities inherent in learning biases. These critiques become especially pertinent when results directly impact human rights and significantly influence people's lives. Yet, there’s a broader issue at play. Historically, humans have used tools to support and refine validation criteria for assessing knowledge, but the mediation between the world and our technology, to understand or imagine it, has always been distinctly human. The advent of machine learning techniques and the digitalization of knowledge resources signal a shift in epistemology, where decisions regarding what to abstract from concrete situations to define categories and concepts for judgment, recognition, and creation are no longer solely within human purview. This shift resembles an industrialization of extraction, with digital data serving as the raw material—concretizing human intelligence and the process of searching, recognizing, and sorting relevant content. While machine learning systems heavily rely on human activities, they perceive these activities as objects or exploitable resources, rather than subjects in the abstraction process.
Teresa Numerico is associate professor of Logic and Philosophy of Science at Università Roma Tre. Her publications include: Alan Turing e l’intelligenza delle macchine, (Franco Angeli, 2006), Web Dragons (Morgan Kaufmann, 2007 with M. Gori and I. Witten), L’umanista digitale (Il Mulino, 2010 with D. Fiormonte e F. Tomasi), The Digital Humanist. A critical inquiry (Punctum 2015), Big Data e algoritmi (Carocci, 2021). She works in the history and philosophy of computer science with a focus on the ethical, political and social issues of the digital age and on the epistemology of digital technologies.