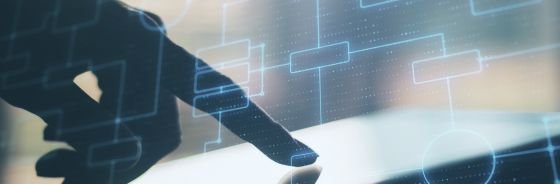
DEIB - Nicola Schiavoni Seminar Room (Building 20)
March 26, 2024 | 12:15 pm
Contacts: Prof. Simone Formentin
Multi-objective optimization problems are ubiquitous in science, engineering, and economics. When dealing with conflicting criteria, there is no single combination of the design variables that can simultaneously achieve the best result for all the objectives, but a set of efficient solutions corresponding to the different trade-offs (Pareto front). Therefore, interaction with the decision-maker is needed to determine the optimal solution aligned with their preferences. In the most common and relevant situations, the decision-maker selects the desired solution among a precomputed set of alternatives, which aim at reproducing the entire spectrum of optimal trade-offs (a posteriori articulation of preferences).
For these reasons, finding an accurate approximation of the Pareto front with as little computational or experimental effort as possible is crucial to ensure the effectiveness and efficiency of the decision-making process. The majority of the existing methods aim at extensively and evenly sampling the Pareto front through the solution of auxiliary optimization problems, to achieve a complete pointwise representation of it. This is impractical when obtaining a single instance of the Pareto front is expensive, for example, requiring time-consuming simulations or experiments. The proposed research aims to develop a new method to estimate the Pareto front, guaranteeing that the worst-case difference between the approximation and the real front lies within a user-defined set after a small number of iterations. The resulting methodology is adopted in the design of innovative decision support systems to help operators control large-scale industrial processes, where balancing production and environmental goals results in a complex challenge.
The seminar is part of the Systems & Control Ph.D. Seminar Series. See the event program for further information.