AI Seminars 2024: The Science and the Engineering of Intelligence
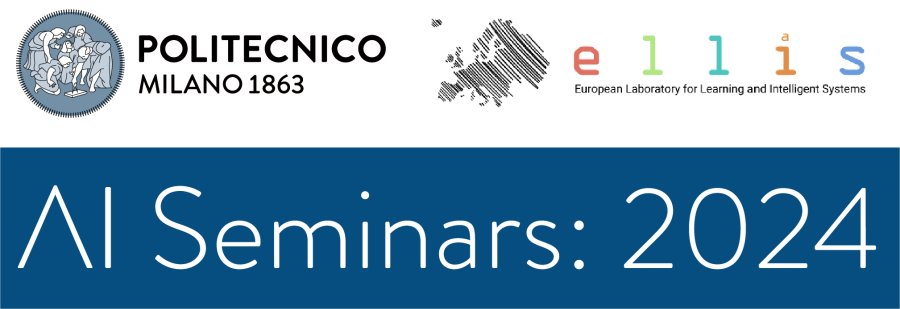
DEIB Seminar Room - Campus Leonardo - Building 20
January 15th, 2024
5.30 pm
Contacts:
Nicola Gatti
Research Line:
Artificial intelligence and robotics
January 15th, 2024
5.30 pm
Contacts:
Nicola Gatti
Research Line:
Artificial intelligence and robotics
Sommario
On January 15th, 2024 at 5.30 pm Tomaso Poggio, Eugene McDermott Professor, Co-Director Center for Brains, Minds and Machines (CBMM) Core founding scientific advisor, MIT Quest for Intelligence - McGovern Institute, CSAIL, Brain Sciences Department (M.I.T.), will deliver the talk titled "The Science and the Engineering of Intelligence" in DEIB Seminar Room (Building 20).
In recent years, artificial intelligence researchers have built impressive systems. Two of my former postdocs — Demis Hassabis and Amnon Shashua — are behind two main recent success stories of AI: AlphaGo and Mobileye, based on two key algorithms, both originally suggested by discoveries in neuroscience: deep learning and reinforcement learning. But now recent engineering advances of the last 4 years — such as transformers, perceivers and MLP mixers— prompt new questions: will science or engineering win the race for AI? Do we need to understand the brain in order to build intelligent machines? or not?
A related question is whether there exist theoretical principles underlying those architectures, including the human brain, that perform so well in learning tasks. A theory of deep learning could solve many of todays problems around AI, such as explainability and control. Though we do not have a full theory as yet, there are very good reasons to believe in the existence of some fundamental principles of learning and intelligence. I will describe one of them which revolves around the curse of dimensionality. Others are about key properties of transformers and LLMs such as ChatGPT. I will argue that in the race for intelligence, understanding fundamental principles of learning and applying them to brains and machines is a compelling and urgent need.
AI Seminars are a series of talks to foster the study of artificial intelligence in Milan.
In recent years, artificial intelligence researchers have built impressive systems. Two of my former postdocs — Demis Hassabis and Amnon Shashua — are behind two main recent success stories of AI: AlphaGo and Mobileye, based on two key algorithms, both originally suggested by discoveries in neuroscience: deep learning and reinforcement learning. But now recent engineering advances of the last 4 years — such as transformers, perceivers and MLP mixers— prompt new questions: will science or engineering win the race for AI? Do we need to understand the brain in order to build intelligent machines? or not?
A related question is whether there exist theoretical principles underlying those architectures, including the human brain, that perform so well in learning tasks. A theory of deep learning could solve many of todays problems around AI, such as explainability and control. Though we do not have a full theory as yet, there are very good reasons to believe in the existence of some fundamental principles of learning and intelligence. I will describe one of them which revolves around the curse of dimensionality. Others are about key properties of transformers and LLMs such as ChatGPT. I will argue that in the race for intelligence, understanding fundamental principles of learning and applying them to brains and machines is a compelling and urgent need.
AI Seminars are a series of talks to foster the study of artificial intelligence in Milan.