Data driven methods for estimating and characterising room acoustic fields
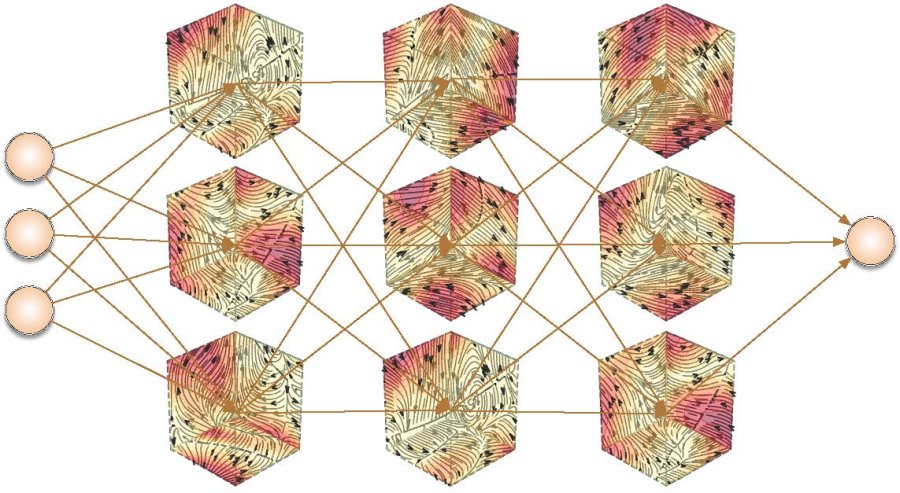
Xenofon Karakonstantis
DTU
DEIB - Seminar Room "N. Schiavoni" (Bld. 20)
November 22nd, 2023
11.00 am
Contacts:
Mirco Pezzoli
Research Line:
Signal processing for multimedia and telecommunications
DTU
DEIB - Seminar Room "N. Schiavoni" (Bld. 20)
November 22nd, 2023
11.00 am
Contacts:
Mirco Pezzoli
Research Line:
Signal processing for multimedia and telecommunications
Sommario
On November 22nd, 2023 at 11.00 am Xenofon Karakonstantis. visiting phd student from DTU, will give a seminar on "Data driven methods for estimating and characterising room acoustic fields" in DEIB Seminar Room "Nicola Schiavoni" (Building 20).
Deep learning strategies have gained prominence in acoustic signal processing, while classical wave equation-based approaches remain prevalent, particularly for sound field reconstruction. Physics-Informed Neural Networks (PINNs) have emerged as a novel framework that integrates data-driven and model-based techniques for solving physical phenomena described by partial differential equations. This work focuses on deep learning based methods for sound field reconstruction with an emphasis on PINN-based reconstruction of arbitrary acoustic fields in three-dimensional space. Apart from outperforming state-of-the-art methods for sound field reconstruction, the presented work is a valuable tool for sound field analysis as it allows for the reconstruction of vectorial quantities such as particle velocity and instantaneous intensity, allowing for a complete characterisation of the sound field.
Deep learning strategies have gained prominence in acoustic signal processing, while classical wave equation-based approaches remain prevalent, particularly for sound field reconstruction. Physics-Informed Neural Networks (PINNs) have emerged as a novel framework that integrates data-driven and model-based techniques for solving physical phenomena described by partial differential equations. This work focuses on deep learning based methods for sound field reconstruction with an emphasis on PINN-based reconstruction of arbitrary acoustic fields in three-dimensional space. Apart from outperforming state-of-the-art methods for sound field reconstruction, the presented work is a valuable tool for sound field analysis as it allows for the reconstruction of vectorial quantities such as particle velocity and instantaneous intensity, allowing for a complete characterisation of the sound field.
Biografia
Xenofon Karakonstantis is a PhD student, researcher, and engineer with expertise in acoustics, signal processing, and sensor array processing. He is currently pursuing a PhD in Data Driven Acoustic Holography at the Technical University of Denmark (DTU), where he is investigating neural generative models for sound field reconstruction. His research interests also include cross-modalities in machine learning and physical models for sound field manipulation, graph neural networks for sound source localization, and acoustic measurements for spatial audio.
Prior to joining DTU, Xenofon held research positions at GN - Jabra and Sennheiser Communications, where he worked on Python DSP applications for audio-related tasks, deep learning framework migration, and higher order ambisonics recording-processing.
He also has experience as an IT specialist and teaching assistant. Currently, he is a visiting PhD in the ISPL of Politecnico di Milano, working on Physics-informed sound field reconstruction.
Prior to joining DTU, Xenofon held research positions at GN - Jabra and Sennheiser Communications, where he worked on Python DSP applications for audio-related tasks, deep learning framework migration, and higher order ambisonics recording-processing.
He also has experience as an IT specialist and teaching assistant. Currently, he is a visiting PhD in the ISPL of Politecnico di Milano, working on Physics-informed sound field reconstruction.