AI Seminars 2023: Augmenting Physics-based Models through Scientific Machine Learning Methods in Computational Medicine
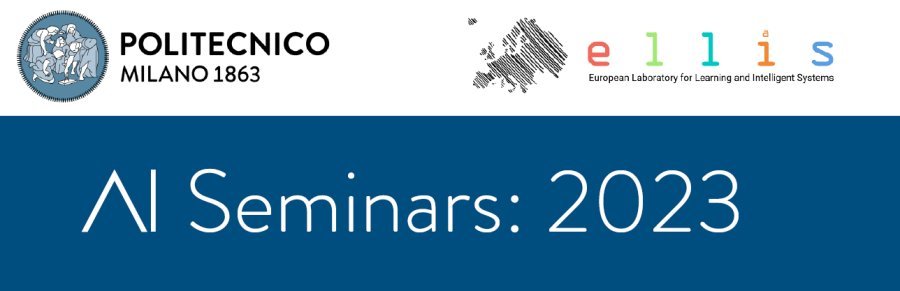
DEIB Conference Room "E. Gatti"
Campus Leonardo - Building 20
September 11th, 2023
5.30 pm
Contacts:
Nicola Gatti
Research Line:
Artificial intelligence and robotics
Campus Leonardo - Building 20
September 11th, 2023
5.30 pm
Contacts:
Nicola Gatti
Research Line:
Artificial intelligence and robotics
Sommario
On September 11th, 2023 at 5.30 pm Stefano Pagani and Francesco Regazzoni will deliver the next seminar of the AI Seminars series on "Augmenting Physics-based Models through Scientific Machine Learning Methods in Computational Medicine" in DEIB Conference Room "Emilio Gatti" (Building 20).
The development of digital twins for patient care is a challenging research field, where the need for accurate responses in short time frames conflicts with the complexity of the underlying physical processes and the large anatomical and functional variability among patients. In this context, physics-based models require long times and computational resources for the numerical discretization of multi-scale and multi-physics differential equation systems, while data-driven methods rarely achieve high accuracy and generalization capabilities. In this talk, we present Scientific Machine Learning (SML) methods that integrate physical knowledge with data-driven techniques to accelerate the evaluation of differential models and address many-query problems - such as sensitivity analysis, data assimilation, optimal control, and uncertainty quantification - in biomedical applications. To speed up input-output evaluations, we develop efficient emulators for problems in variable domains by exploiting the approximation power of neural networks. In addition, we construct deep learning models that encode differential models directly into the architectures by employing dimensionality reduction techniques or by adding physics-informed penalty terms to the loss function.
The development of digital twins for patient care is a challenging research field, where the need for accurate responses in short time frames conflicts with the complexity of the underlying physical processes and the large anatomical and functional variability among patients. In this context, physics-based models require long times and computational resources for the numerical discretization of multi-scale and multi-physics differential equation systems, while data-driven methods rarely achieve high accuracy and generalization capabilities. In this talk, we present Scientific Machine Learning (SML) methods that integrate physical knowledge with data-driven techniques to accelerate the evaluation of differential models and address many-query problems - such as sensitivity analysis, data assimilation, optimal control, and uncertainty quantification - in biomedical applications. To speed up input-output evaluations, we develop efficient emulators for problems in variable domains by exploiting the approximation power of neural networks. In addition, we construct deep learning models that encode differential models directly into the architectures by employing dimensionality reduction techniques or by adding physics-informed penalty terms to the loss function.
Numerical results show that, even in scenarios characterized by incomplete or noisy data, these SML methods improve the efficiency and accuracy of approximating quantities of interest or solving inverse or parameter estimation problems that are pivotal for decision-making.
AI Seminars are a series of talks to foster the study of artificial intelligence in Milan.
An aperitif will be served after the seminar.