Combining memristors and brain-inspired principles to build more efficient computing systems
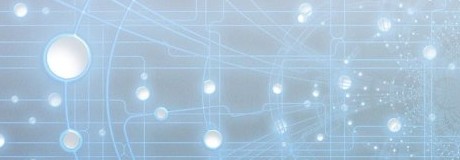
John Paul Strachan
Peter Grünberg Institute (PGI-14), Forschungszentrum Jülich, Jülich, Germany and RWTH Aachen University, Aachen, Germany
DEIB - "Schiavoni" Seminar Room (Bld. 20)
September 5th, 2023
3.30 pm
Contacts:
Daniele Ielmini
Research Line:
Electron devices
Peter Grünberg Institute (PGI-14), Forschungszentrum Jülich, Jülich, Germany and RWTH Aachen University, Aachen, Germany
DEIB - "Schiavoni" Seminar Room (Bld. 20)
September 5th, 2023
3.30 pm
Contacts:
Daniele Ielmini
Research Line:
Electron devices
Sommario
On September 5th, 2023 Dr John Paul Strachan, director of Peter Grünberg Institute (PGI-14), Forschungszentrum Jülich, Jülich, Germany and RWTH Aachen University (Aachen, Germany) will give a talk on "Combining memristors and brain-inspired principles to build more efficient computing systems" in Deib Seminar Room.
Simultaneously today, there is interest in building more efficient computing hardware for challenging workloads (AI, machine learning, optimization, etc.), as well as a drive to overhaul the von Neumann architecture toward more brain-like architectures. I will attempt to cover these two topics, with the theme that both goals involve revamping today’s memory systems and inventing in-memory computing architectures. One approach has been exploring “associative memory” circuits, such as Content-Addressable Memories (CAM) as a core in-memory computing block. CAM circuits allow for complex pattern storage and rapid access, but capacities are highly limited in size and consume much power. We show that the use of non-volatile and analog memristive devices enable CAM circuits of higher data density and lower energy than CMOS-only CAM circuits. We further use them directly in a variety of computing applications, including security, genomics, and machine learning. Going further, we are interested in how “learning” might be incorporated into such memory circuits and I describe a modified “differentiable” CAM circuit that is compatible with gradient-based training algorithms and illustrate some applications of such a circuit. Lastly, I would like to discuss the challenging computing area of combinatorial optimization, and how in-memory and analog computing offer potential speed-up and energy efficiency. I will describe our proposed hardware and algorithmic approach that is based on mixed analog-digital memristor-based circuits implementing Hopfield neural network dynamics. We augment Hopfield networks to support higher order interactions, as needed for many problem classes, as well as constraint enforcement. I will describe our prototype systems, performance, and comparisons to the state-of-the-art.
Simultaneously today, there is interest in building more efficient computing hardware for challenging workloads (AI, machine learning, optimization, etc.), as well as a drive to overhaul the von Neumann architecture toward more brain-like architectures. I will attempt to cover these two topics, with the theme that both goals involve revamping today’s memory systems and inventing in-memory computing architectures. One approach has been exploring “associative memory” circuits, such as Content-Addressable Memories (CAM) as a core in-memory computing block. CAM circuits allow for complex pattern storage and rapid access, but capacities are highly limited in size and consume much power. We show that the use of non-volatile and analog memristive devices enable CAM circuits of higher data density and lower energy than CMOS-only CAM circuits. We further use them directly in a variety of computing applications, including security, genomics, and machine learning. Going further, we are interested in how “learning” might be incorporated into such memory circuits and I describe a modified “differentiable” CAM circuit that is compatible with gradient-based training algorithms and illustrate some applications of such a circuit. Lastly, I would like to discuss the challenging computing area of combinatorial optimization, and how in-memory and analog computing offer potential speed-up and energy efficiency. I will describe our proposed hardware and algorithmic approach that is based on mixed analog-digital memristor-based circuits implementing Hopfield neural network dynamics. We augment Hopfield networks to support higher order interactions, as needed for many problem classes, as well as constraint enforcement. I will describe our prototype systems, performance, and comparisons to the state-of-the-art.
Biografia
John Paul Strachan directs the Peter Grünberg Institute on Neuromorphic Compute Nodes (PGI-14) at Forschungszentrum Jülich and is a Professor at RWTH Aachen. Previously he led the Emerging Accelerators team as a Distinguished Technologist at Hewlett Packard Labs, HPE. His teams explore novel types of hardware accelerators using emerging device technologies, with expertise spanning materials, device physics, circuits, architectures, benchmarking and building prototype systems. Their interests span applications in machine learning, network security, and optimization. John Paul has degrees in physics and electrical engineering from MIT and a PhD in applied physics from Stanford University. He has over 60 patents, has authored or co-authored over 100 peer-reviewed papers, and been the PI in many USG research grants. He has previously worked on nanomagnetic devices for memory for which he was awarded the Falicov Award from the American Vacuum Society, and has developed sensing systems for precision agriculture in a company which he co-founded. He serves in professional societies including IEEE IEDM ExComm, the Nanotechnology Council ExComm, and past program chair and steering member of the International Conference on Rebooting Computing.