Identification of switched systems based on the randomized algorithm
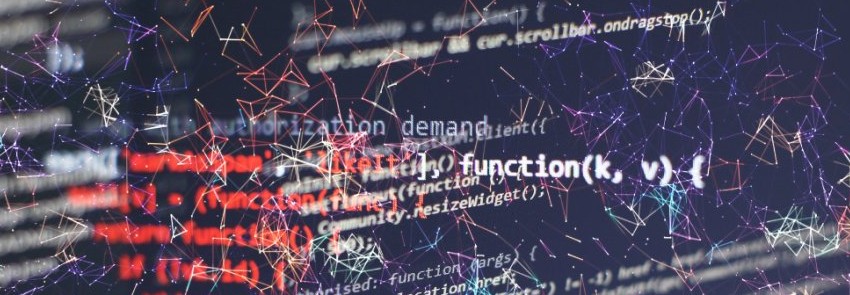
Miao Yu
DEIB PHD Student
DEIB - Conference Room "E. Gatti" (Building 20)
July 10th, 2023
12.10 pm
Contacts:
Lorenzo Fagiano
Simone Formentin
Research Line:
Control systems
DEIB PHD Student
DEIB - Conference Room "E. Gatti" (Building 20)
July 10th, 2023
12.10 pm
Contacts:
Lorenzo Fagiano
Simone Formentin
Research Line:
Control systems
Sommario
On July 10th, 2023 at 12.10 pm Miao Yu, DEIB PHD Student, will give a seminar on "Identification of switched systems based on the randomized algorithm" in DEIB Conference Room.
The identification of switched systems is a complex optimization problem that involves both continuous (parametrizations of the local models, a.k.a. modes) and discrete variables (model structures, switching signal). In particular, the combinatorial complexity associated with the estimation of the switching signal grows exponentially with the number of samples, which makes data segmentation (i.e. estimating the number and location of mode switchings, and the mode sequence) a challenging problem. In this context, I will introduce the work that extends a previously developed randomized approach for the identification of switched systems to encompass the estimation of the switching locations. The method operates by extracting samples from a probability distribution of switched models, and gathering information from the associated model performances to update the distribution, until convergence to a limit distribution associated to a specific model. A suitable probability distribution is employed to represent the likelihood of a mode switching at a certain time, and the update process is designed to correct the switching locations and remove redundant switchings.
The identification of switched systems is a complex optimization problem that involves both continuous (parametrizations of the local models, a.k.a. modes) and discrete variables (model structures, switching signal). In particular, the combinatorial complexity associated with the estimation of the switching signal grows exponentially with the number of samples, which makes data segmentation (i.e. estimating the number and location of mode switchings, and the mode sequence) a challenging problem. In this context, I will introduce the work that extends a previously developed randomized approach for the identification of switched systems to encompass the estimation of the switching locations. The method operates by extracting samples from a probability distribution of switched models, and gathering information from the associated model performances to update the distribution, until convergence to a limit distribution associated to a specific model. A suitable probability distribution is employed to represent the likelihood of a mode switching at a certain time, and the update process is designed to correct the switching locations and remove redundant switchings.