AI Seminars 2023: Recent advancements in no-regret dynamics
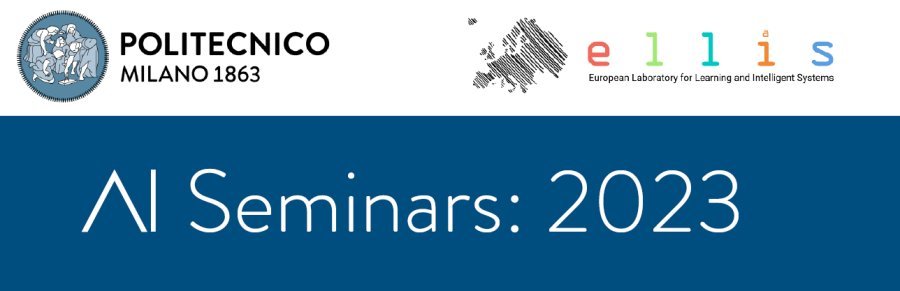
DEIB Conference Room "E. Gatti"
Campus Leonardo - Building 20
July 13th, 2023
5.30 pm
Contacts:
Nicola Gatti
Research Line:
Artificial intelligence and robotics
Campus Leonardo - Building 20
July 13th, 2023
5.30 pm
Contacts:
Nicola Gatti
Research Line:
Artificial intelligence and robotics
Sommario
On July 13th, 2023 at 5.30 pm Martino Bernasconi (DEIB, PHD Student in Information Technology) and Federico Cacciamani (DEIB, PHD Student in Information Technology), will deliver the next seminar of the AI Seminars series on "Recent advancements in no-regret dynamics" in DEIB Conference Room "Emilio Gatti" (Building 20).
Learning to persuade in sequential decision-making - Martino Bernasconi.
We present a generalization of the information design problem, where an informed sender tries to influence the behavior of a self-interested receiver. We generalize the decision problem faced by the receiver to a sequential decision-making (SDM) problem and consider a sequential interaction between the SDM and the receiver over T rounds. At each round, the sender observes the realizations of random events in the SDM problem. This begets the challenge of how to incrementally disclose such information to the receiver to persuade them to follow (desirable) action recommendations. We present a learning framework in which the nature's parameter in the SDM are unknown to both the sender and the receiver, and, thus, they have to gradually learn them while persuading the receiver. We provide strong negative result: no algorithm can be persuasive. Thus, we relax persuasiveness requirements by focusing on algorithms that guarantee that the receiver's regret in following recommendations grows sub-linearly. Then we provide a O(sqrt(T)) regret for both sender and the receiver, while we provide a regret frontier in the partial feedback case, that matches a parametric lower bound on most of the frontier.
Mechanism design for information acquisition - Federico Cacciamani.
Most modern decision-making scenarios are characterized by an increasing spread of information across multiple sources, making it crucial to study how to accurately gather such information. In this talk, we explore the problem of information acquisition from a mechanism design perspective. Our model encompasses a decision maker (called principal) that interacts with a set of agents holding some information on an underlying state of nature. The agents can communicate (either truthfully or not) their information to the principal, which, based on the information received, takes action and can pay the agents. We study the online learning problem faced by a principal that does not have knowledge of the game and show how such an uninformed principal can interact with the agents in order to efficiently learn an optimal mechanism.
AI Seminars are a series of talks to foster the study of artificial intelligence in Milan.
An aperitif will be served after the seminar.
Please register here.
Learning to persuade in sequential decision-making - Martino Bernasconi.
We present a generalization of the information design problem, where an informed sender tries to influence the behavior of a self-interested receiver. We generalize the decision problem faced by the receiver to a sequential decision-making (SDM) problem and consider a sequential interaction between the SDM and the receiver over T rounds. At each round, the sender observes the realizations of random events in the SDM problem. This begets the challenge of how to incrementally disclose such information to the receiver to persuade them to follow (desirable) action recommendations. We present a learning framework in which the nature's parameter in the SDM are unknown to both the sender and the receiver, and, thus, they have to gradually learn them while persuading the receiver. We provide strong negative result: no algorithm can be persuasive. Thus, we relax persuasiveness requirements by focusing on algorithms that guarantee that the receiver's regret in following recommendations grows sub-linearly. Then we provide a O(sqrt(T)) regret for both sender and the receiver, while we provide a regret frontier in the partial feedback case, that matches a parametric lower bound on most of the frontier.
Mechanism design for information acquisition - Federico Cacciamani.
Most modern decision-making scenarios are characterized by an increasing spread of information across multiple sources, making it crucial to study how to accurately gather such information. In this talk, we explore the problem of information acquisition from a mechanism design perspective. Our model encompasses a decision maker (called principal) that interacts with a set of agents holding some information on an underlying state of nature. The agents can communicate (either truthfully or not) their information to the principal, which, based on the information received, takes action and can pay the agents. We study the online learning problem faced by a principal that does not have knowledge of the game and show how such an uninformed principal can interact with the agents in order to efficiently learn an optimal mechanism.
AI Seminars are a series of talks to foster the study of artificial intelligence in Milan.
An aperitif will be served after the seminar.
Please register here.