Cost-aware distributionally robust optimization with out-of-sample performance guarantees
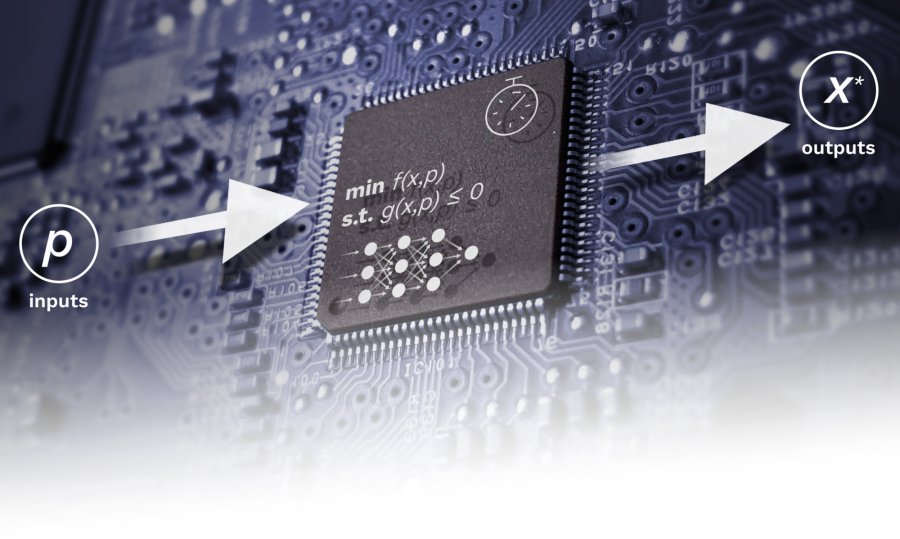
Prof. Panagiotis (Panos) Patrinos
Department of Electrical Engineering (ESAT)
KU Leuven, Belgium
DEIB - Conference Room "E. Gatti" (Bld. 20)
June 23rd, 2023
4.00 pm
Contacts:
Lorenzo Fagiano
Research Line:
Control systems
Department of Electrical Engineering (ESAT)
KU Leuven, Belgium
DEIB - Conference Room "E. Gatti" (Bld. 20)
June 23rd, 2023
4.00 pm
Contacts:
Lorenzo Fagiano
Research Line:
Control systems
Sommario
On June 23rd, 2023 at 4.00 pm Panagiotis (Panos) Patrinos, associate professor at the Department of Electrical Engineering (ESAT) of KU Leuven, Belgium. will hold a seminar on "Cost-aware distributionally robust optimization with out-of-sample performance guarantees" in DEIB Conference Room (Building 20).
Motivated by recent successes, machine learning is achieving widespread adoption at increasingly fast rates. However, current machine learning techniques still provide few guarantees regarding correctness and/or safety. This drawback can be partially mitigated by the ever-increasing sample sizes, but this is not a valid solution in all application domains, e.g., control of safety-critical systems, where typically, stronger guarantees are required, computational hardware is limited, and data may inherently be scarce. Our aim is therefore to develop machine learning approaches with provable finite-sample guarantees. Distributionally robust optimization has proven to be a useful tool in this respect, although current formulations still suffer from excessive conservatism. To address this, we present novel distributionally robust formulations for stochastic programming that exploit the structure of the cost function in order to obtain significantly tighter mean bounds. We show empirically that this results in improved performance while maintaining guarantees on out-of-sample performance.
Motivated by recent successes, machine learning is achieving widespread adoption at increasingly fast rates. However, current machine learning techniques still provide few guarantees regarding correctness and/or safety. This drawback can be partially mitigated by the ever-increasing sample sizes, but this is not a valid solution in all application domains, e.g., control of safety-critical systems, where typically, stronger guarantees are required, computational hardware is limited, and data may inherently be scarce. Our aim is therefore to develop machine learning approaches with provable finite-sample guarantees. Distributionally robust optimization has proven to be a useful tool in this respect, although current formulations still suffer from excessive conservatism. To address this, we present novel distributionally robust formulations for stochastic programming that exploit the structure of the cost function in order to obtain significantly tighter mean bounds. We show empirically that this results in improved performance while maintaining guarantees on out-of-sample performance.
Biografia
Panagiotis (Panos) Patrinos is associate professor at the Department of Electrical Engineering (ESAT) of KU Leuven, Belgium. In 2014 he was a visiting professor at Stanford.
He received his PhD in Control and Optimization, M.S. in Applied Mathematics and M.Eng. from the National Technical University of Athens in 2010, 2005 and 2003, respectively.
After his PhD he held postdoc positions at the University of Trento and IMT Lucca, Italy, where he became an assistant professor in 2012. His current research interests are in the theory and algorithms of structured convex and nonconvex optimization and predictive control with a focus on large-scale, distributed, stochastic and embedded optimization and a wide range of application areas including automotive, aerospace, machine learning, signal processing and energy.
This seminar is organized in the framework of the European Project ELO-X, https://elo-x.eu/
He received his PhD in Control and Optimization, M.S. in Applied Mathematics and M.Eng. from the National Technical University of Athens in 2010, 2005 and 2003, respectively.
After his PhD he held postdoc positions at the University of Trento and IMT Lucca, Italy, where he became an assistant professor in 2012. His current research interests are in the theory and algorithms of structured convex and nonconvex optimization and predictive control with a focus on large-scale, distributed, stochastic and embedded optimization and a wide range of application areas including automotive, aerospace, machine learning, signal processing and energy.
This seminar is organized in the framework of the European Project ELO-X, https://elo-x.eu/