Physics enhanced Artificial Intelligence for Industrial Process Control
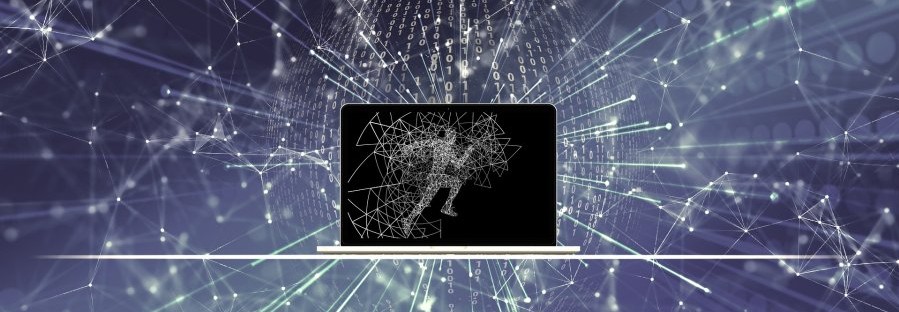
Marco Leonesio
PHD Student
DEIB - Conference Room "E. Gatti" (Building 20)
April 13th, 2023
11.50 am
Contacts:
Simone Formentin
Research Line:
Control systems
PHD Student
DEIB - Conference Room "E. Gatti" (Building 20)
April 13th, 2023
11.50 am
Contacts:
Simone Formentin
Research Line:
Control systems
Sommario
On April 13th, 2023 at 11.50 am Marco Leonesio, PHD Student in Information Technology, will give a seminar on "Physics enhanced Artificial Intelligence for Industrial Process Control" in DEIB Conference Room.
The current manufacturing environment places a growing demand on autonomous control and optimization of manufacturing processes, especially in unattended machines. Nowadays, process optimization strategies based on Artificial Intelligence (AI) have not reached relevant exploitation at the machines level (except for robotic applications), despite the appropriate amount of research carried out on this topic. Indeed, pure AI strategies present some drawbacks. They need a consistent amount of data to train the system; therefore, they are not immediately effective. They ignore the treasury of a-priori knowledge accumulated by long-term physics-based modeling activity and technologists' experience. Moreover, the machine learning models need to be properly chosen ad adapted to the particular application. Finally, AI often generates distrust due to a lack of interpretability. The proposed research seeks the integration of domain-knowledge in Machine Learning optimization techniques to: reduce the training effort; preserve the physic-based core of the control architecture in order to make the actions interpretable by experts; develop methods and interface to continuously improve control performance by a collection of sporadic expert judgments and/or new training data entries.
The current manufacturing environment places a growing demand on autonomous control and optimization of manufacturing processes, especially in unattended machines. Nowadays, process optimization strategies based on Artificial Intelligence (AI) have not reached relevant exploitation at the machines level (except for robotic applications), despite the appropriate amount of research carried out on this topic. Indeed, pure AI strategies present some drawbacks. They need a consistent amount of data to train the system; therefore, they are not immediately effective. They ignore the treasury of a-priori knowledge accumulated by long-term physics-based modeling activity and technologists' experience. Moreover, the machine learning models need to be properly chosen ad adapted to the particular application. Finally, AI often generates distrust due to a lack of interpretability. The proposed research seeks the integration of domain-knowledge in Machine Learning optimization techniques to: reduce the training effort; preserve the physic-based core of the control architecture in order to make the actions interpretable by experts; develop methods and interface to continuously improve control performance by a collection of sporadic expert judgments and/or new training data entries.