Direct data-driven control in vehicle applications
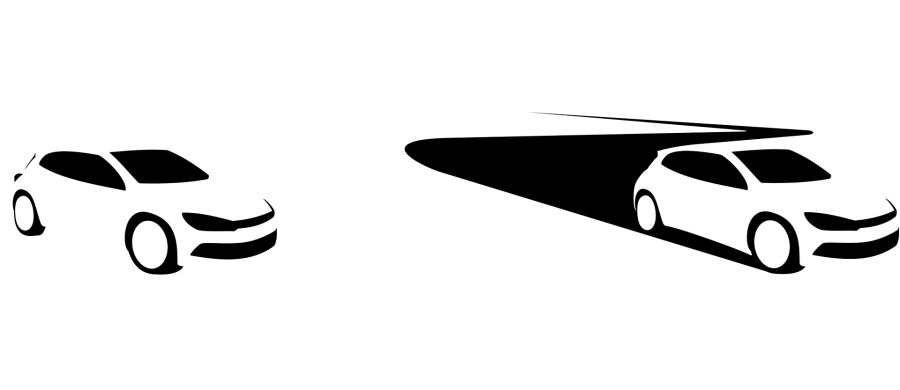
Edoardo Catenaro
PHD Student
DEIB - Conference Room "E. Gatti" (Building 20)
February 13th, 2023
11.30 am
Contacts:
Simone Formentin
Research Line:
Control systems
PHD Student
DEIB - Conference Room "E. Gatti" (Building 20)
February 13th, 2023
11.30 am
Contacts:
Simone Formentin
Research Line:
Control systems
Sommario
On February 13th, 2023 at 11.30 am Edoardo Catenaro, PHD Student in Information Technology, will give a seminar on "Direct data-driven control in vehicle applications" in DEIB Conference Room.
This PhD project investigates optimal calibration frameworks for direct-control in vehicle applications. The problem is formulated as a black-box global optimization task that aims at minimizing an objective function (OF). To tackle this issue data-driven methods rely on available measured data to spot connections and correlations among system’s variables, guiding the optimization towards the best controller candidate. Among the wide spectrum of applications, model-free approaches have recently found interest in automotive field, where modeling cost in terms of parameters identification and uncertainty are non-negligible. To overcome these issues data-driven strategies leverage the advantages of using more traditional control techniques, which have been proved to be effective in a nominal scenario, but provide an experiment-driven procedure for their parameters calibration, aiming at a faster and robust tuning. Based on the aforementioned calibration framework, the proposed work develops in two branches. The first deals with those direct-control problems where certain calibration tunings affect the closed-loop stability, thus producing potential system failures. The second addresses black-box optimization problems where the OF is hardly quantifiable and can only be assessed by a human decision maker, thus relying on preference-based algorithm rather than performance-based. The presented optimization frameworks are capable of automatically providing the controller parameters, yielding the target closed-loop performance, while coping with the limitations imposed by the problem at hand.
This PhD project investigates optimal calibration frameworks for direct-control in vehicle applications. The problem is formulated as a black-box global optimization task that aims at minimizing an objective function (OF). To tackle this issue data-driven methods rely on available measured data to spot connections and correlations among system’s variables, guiding the optimization towards the best controller candidate. Among the wide spectrum of applications, model-free approaches have recently found interest in automotive field, where modeling cost in terms of parameters identification and uncertainty are non-negligible. To overcome these issues data-driven strategies leverage the advantages of using more traditional control techniques, which have been proved to be effective in a nominal scenario, but provide an experiment-driven procedure for their parameters calibration, aiming at a faster and robust tuning. Based on the aforementioned calibration framework, the proposed work develops in two branches. The first deals with those direct-control problems where certain calibration tunings affect the closed-loop stability, thus producing potential system failures. The second addresses black-box optimization problems where the OF is hardly quantifiable and can only be assessed by a human decision maker, thus relying on preference-based algorithm rather than performance-based. The presented optimization frameworks are capable of automatically providing the controller parameters, yielding the target closed-loop performance, while coping with the limitations imposed by the problem at hand.