AI Seminars 2023: On the learning landscape of deep and recurrent neural networks
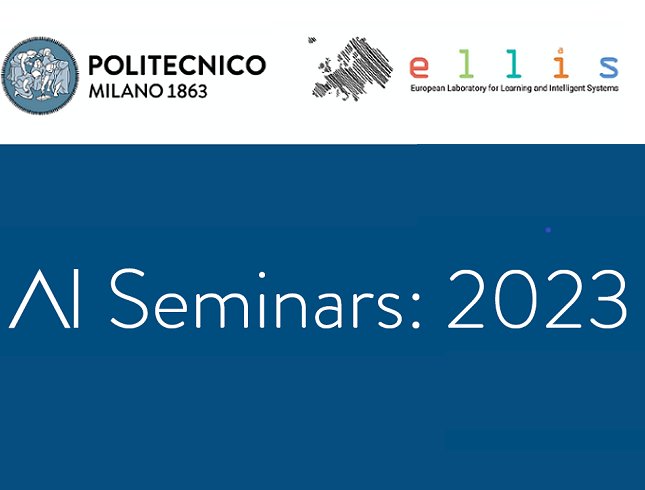
DEIB - Conference Room "E. Gatti" (Bldg. 20)
January 24th, 2023
5.30 pm
Contacts:
Nicola Gatti
Research Line:
Artificial intelligence and robotics
January 24th, 2023
5.30 pm
Contacts:
Nicola Gatti
Research Line:
Artificial intelligence and robotics
Sommario
In the framework of the AI Seminars 2023, on January 24th, 2023 at 5.30 pm, the seminar titled "On the learning landscape of deep and recurrent neural networks" will be held by Riccardo Zecchina, Full Professor at Università Bocconi di Milano, in DEIB Conference Room.
In this talk, we will discuss the geometrical structure of the space of solutions (zero error configurations) in overparametrized non-convex neural networks when trained to classify patterns taken from some natural distribution.
Building on statistical physics techniques for the study of disordered systems, we analyze the geometric structure of the different minima and critical points of the error loss function as the number of parameters increases and we relate this to learning performance.
Of particular interest is the role of rare flat minima which are both accessible to algorithms and have good generalisation properties, on the contrary to dominating minima which are almost impossible to sample. We will show that the appearance of rare flat minima defines a phase boundary at which algorithms start to find solutions efficiently.
In this talk, we will discuss the geometrical structure of the space of solutions (zero error configurations) in overparametrized non-convex neural networks when trained to classify patterns taken from some natural distribution.
Building on statistical physics techniques for the study of disordered systems, we analyze the geometric structure of the different minima and critical points of the error loss function as the number of parameters increases and we relate this to learning performance.
Of particular interest is the role of rare flat minima which are both accessible to algorithms and have good generalisation properties, on the contrary to dominating minima which are almost impossible to sample. We will show that the appearance of rare flat minima defines a phase boundary at which algorithms start to find solutions efficiently.