AI Seminars 2023: Deep Learning & Scientific Computing: challenges and perspectives in reduced order modeling of physical systems
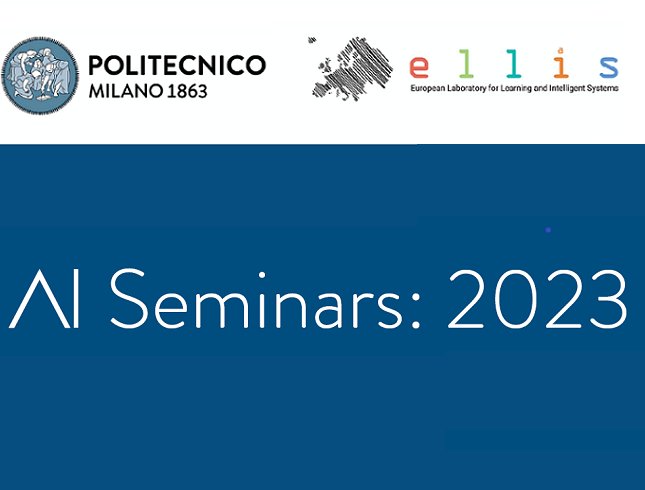
DEIB - Conference Room "E. Gatti" (Bldg. 20)
January 10th, 2023
5.30 pm
Contacts:
Nicola Gatti
Research Line:
Artificial intelligence and robotics
January 10th, 2023
5.30 pm
Contacts:
Nicola Gatti
Research Line:
Artificial intelligence and robotics
Sommario
In the framework of the AI Seminars 2023, on January 10th, 2023 at 5.30 pm, the seminar titled "Deep Learning & Scientific Computing: challenges and perspectives in reduced order modeling of physical systems" will be held by Andrea Manzoni, Associate Professor at DMAT Politecnico di Milano, in DEIB Conference Room.
In the last few years, machine learning - in particular deep learning - algorithms and tools have been increasingly exploited to perform, enhance and accelerate computer simulations of mathematical models in applied sciences and engineering. This seminar aims at showing recent results obtained in the general area of deep learning in scientific computing, with an emphasis on the application of deep learning algorithms for the construction of efficient, yet accurate, reduced order models for parametrized differential problems. Real-time numerical simulation of distributed systems, as well as multi-fidelity strategies leveraging on outputs of models with different level of complexity and sparse identification of large-scale nonlinear dynamical systems, are few instances of problems that will be taken into account, along with several applications ranging from fluid dynamics to structural mechanics.
In the last few years, machine learning - in particular deep learning - algorithms and tools have been increasingly exploited to perform, enhance and accelerate computer simulations of mathematical models in applied sciences and engineering. This seminar aims at showing recent results obtained in the general area of deep learning in scientific computing, with an emphasis on the application of deep learning algorithms for the construction of efficient, yet accurate, reduced order models for parametrized differential problems. Real-time numerical simulation of distributed systems, as well as multi-fidelity strategies leveraging on outputs of models with different level of complexity and sparse identification of large-scale nonlinear dynamical systems, are few instances of problems that will be taken into account, along with several applications ranging from fluid dynamics to structural mechanics.